In today's digital age, we are increasingly relying on artificial intelligence (AI) to perform a myriad of tasks, make complex decisions, and even interact with us on a social and personal level. As a result of this growing dependency, it becomes paramount that we take into account the ethical implications of this powerful technology. One of the most significant ethical dilemmas that we face in the realm of AI today is the issue of bias.
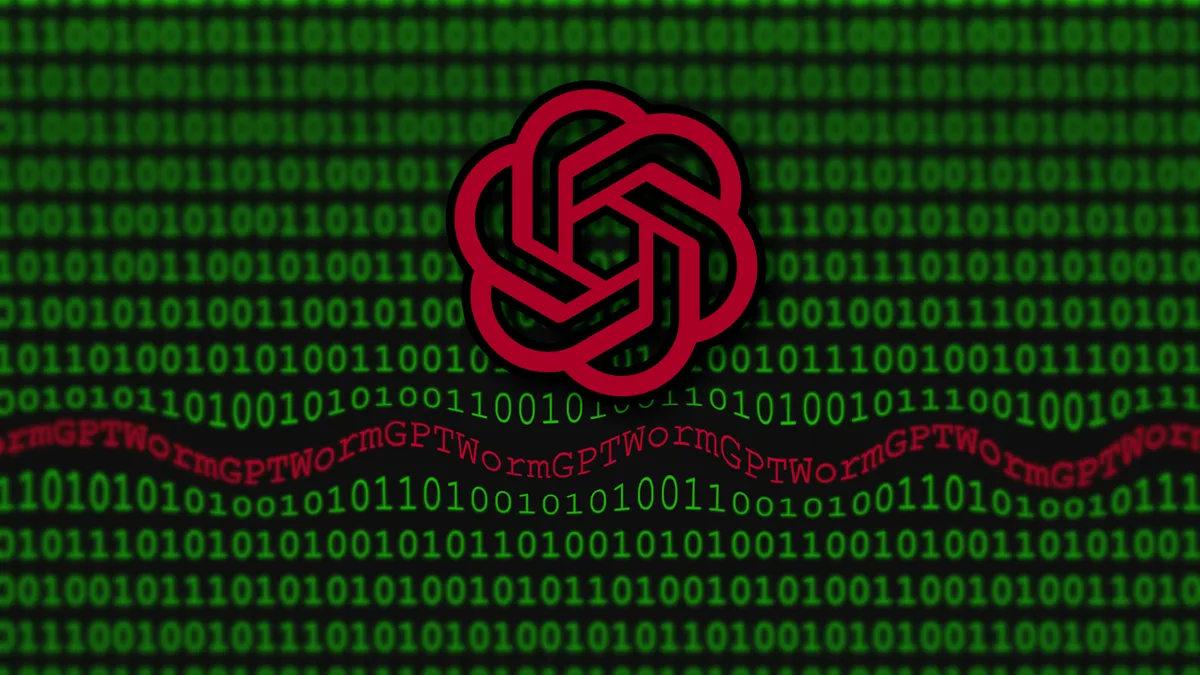
Bias in AI can take on many forms and manifest in various ways. It can range from an AI chatbot that uses discriminatory language, to a sophisticated AI hiring tool that inadvertently favors certain demographics over others. This bias usually stems from the data the AI was trained on. If the training data contains biased information, the AI will not only reproduce this bias, but there is a high probability that it will amplify this bias, creating a cycle that can be difficult to break.
Suggestions for Addressing AI Bias
- Diversify the AI team: Having a diverse team of AI developers can help to prevent unconscious biases from creeping into the AI. This means hiring people of different genders, ethnicities, backgrounds, and perspectives.
- Use inclusive data: Ensure that the datasets used to train the AI are as inclusive and diverse as possible. This will likely involve sourcing data from a variety of different places and ensuring that the data accurately represents the diversity of the population the AI will serve.
- Regular audits: Conduct regular audits of the AI's decisions to detect any patterns of bias. This should involve both quantitative methods (such as statistical tests) and qualitative methods (such as user interviews).
- Transparency and explainability: Make the AI's decision-making process as transparent and explainable as possible. This may involve using techniques such as "explainable AI" (XAI) to make the AI's decisions understandable to humans.
- Public input: Involve the public in decisions about how the AI should work. This might include public consultations or surveys about how the AI should make decisions and handle trade-offs between different ethical principles.
Using OpenAI's GPT-3 as a Case Study
To further illustrate this point, let's delve into the workings of OpenAI's GPT-3, a state-of-the-art language processing AI. GPT-3 has been trained on an extensive and diverse range of internet text. However, due to the inherent bias in the internet text it was trained on, GPT-3 can sometimes produce outputs that are skewed, thus providing us with a clear example of how biased training data can lead to bias in the AI's outputs.
The Far-Reaching Impact of Bias
Bias in AI can have far-reaching impacts that extend beyond just the users of the technology. These impacts can permeate various sectors of society, including employment, education, healthcare, and criminal justice. For instance, in the job market, an AI hiring tool that is biased against certain demographics could lead to unfair hiring practices and workplace discrimination. In the education sector, AI tools used for college admissions or standardized testing could disadvantage certain groups of students. In healthcare, AI diagnostic tools could provide inaccurate diagnoses or treatments for certain populations. In the criminal justice system, AI risk assessment tools could lead to unjust sentencing or bail decisions. These are just a few examples, but they clearly illustrate how AI bias can propagate and amplify societal inequities. Therefore, it is crucial to address these biases to prevent harm and ensure fairness and justice.
The Challenge of Mitigating AI Bias
Mitigating bias in AI is a challenging task, but it is also a necessary endeavor if we are to ensure fairness and equity. This process involves making certain that the training data is as unbiased and representative as possible. It also involves regularly auditing AI outputs for any signs of bias and making necessary adjustments when these biases are detected.
Steps to detect biased AI model
- Analyze the Training Data: The first step in detecting bias is to analyze the training data. Look for any significant imbalances or patterns that could lead to bias.
- Test the AI on a Varied Set of Data: After the AI has been trained, test it on a varied set of data that is different from the training data. This will help reveal any biases that may not have been obvious during training.
- Audit the AI's Decisions: Audit the decisions made by the AI. Look for patterns in the decisions that suggest bias, such as consistently favoring one group over another.
- Use Bias Detection Tools: There are various tools available that can help to detect bias in AI models. These tools can analyze the AI's decisions and highlight potential areas of bias.
- Solicit Feedback from Users: Users can often provide valuable insights on whether or not the AI is demonstrating bias. Regularly solicit and listen to their feedback.
For instance, consider an AI system developed to screen resumes in the hiring process. If the AI was trained on a dataset primarily consisting of resumes from male candidates in the tech industry, it may inadvertently favor resumes that mirror those in its training data. This could result in the system downgrading resumes from female candidates or candidates from other industries who may be equally or more qualified for the position. This serves to illustrate how biases in training data can lead to unfair outcomes in AI applications.